Foreword
In this article, we will demonstrate how to use PyTorch to recognize simple numeric graphic CAPTCHAs
Environment Preparation
Install dependency packages
conda install pytorch torchvision torchaudio cpuonly -c pytorch
sudo apt-get install libgl1 # for opencv
pip install requests matplotlib opencv-python
Download CAPTCHA images (we return the CAPTCHA value in the HTTP header for easy annotation of the original dataset):
CAPTCHA_URL = 'https://captcha.tomo.wang'
r = requests.get(CAPTCHA_URL)
captcha = r.headers['X-Captcha']
with open('{}.png'.format(captcha), 'wb') as f:
f.write(r.content)
Image Processing and Training
Preparation
First, we import the necessary packages
import os
import re
import sys
import argparse
import glob
from io import BytesIO
import requests
import numpy as np
import cv2
import matplotlib.pyplot as plt
import torch
from torch.autograd import Variable
import torch.nn.functional as F
Define relevant constants for program execution
- Number of characters
- CAPTCHA width and height
- Cropped character width and height
- …
NUM_CHARS = 4
CAPTCHA_WIDTH = 200
CAPTCHA_HEIGHT = 62
CH_WIDTH = 20
CH_HEIGHT = 28
CAPTCHA_DIR = './images'
TORCH_NET_PATH = 'captcha.torch'
BG_COLOR = (243, 251, 254) # captcha backgroud color
BG_THRESHOLD = 245
BLANK_THRESHHOLD = 1
DOTS_THRESHOLD = 3
CH_MIN_WIDTH = 8
Obtaining and Displaying CAPTCHA Images
def get_captcha():
CAPTCHA_URL = 'https://captcha.tomo.wang'
r = requests.get(CAPTCHA_URL)
return r.content
img = get_captcha()
plt.imshow(plt.imread(BytesIO(img)))
<matplotlib.image.AxesImage at 0x7f1fcc4d7c40>
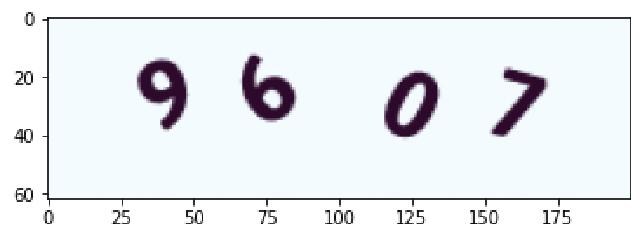
A CAPTCHA image contains a background and characters of different colors, with a total of four characters. Before training, it needs to be grayed out and segmented.
img_array = np.asarray(bytearray(img), dtype=np.uint8)
img = cv2.imdecode(img_array, cv2.IMREAD_GRAYSCALE)
assert img.shape == (CAPTCHA_HEIGHT, CAPTCHA_WIDTH)
img = cv2.threshold(img, BG_THRESHOLD, 255, cv2.THRESH_BINARY_INV)[1]
plt.imshow(cv2.cvtColor(img, cv2.COLOR_GRAY2BGR))
<matplotlib.image.AxesImage at 0x7f1fcc3e7e80>
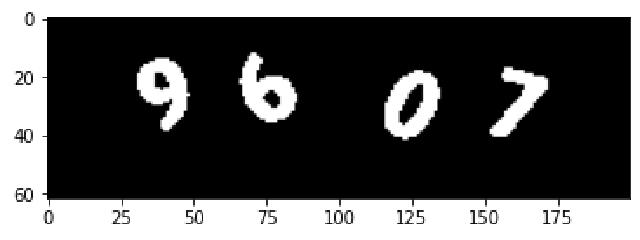
Segmentation
Cutting the image into four independent characters
def _denoise(img):
img = cv2.threshold(img, BG_THRESHOLD, 255, cv2.THRESH_BINARY_INV)[1]
return img
def _preprocess(img):
img = img.copy()
img = _denoise(img)
return img
def find_filled_row(rows):
for i, row in enumerate(rows):
dots = np.sum(row) // 255
if dots >= DOTS_THRESHOLD:
return i
assert False, 'cannot find filled row'
def pad_ch(ch):
pad_w = CH_WIDTH - ch.shape[1]
assert pad_w >= 0, 'bad char width'
pad_w1 = pad_w // 2
pad_w2 = pad_w - pad_w1
pad_h = CH_HEIGHT - ch.shape[0]
assert pad_h >= 0, 'bad char height'
pad_h1 = pad_h // 2
pad_h2 = pad_h - pad_h1
return np.pad(ch, ((pad_h1, pad_h2), (pad_w1, pad_w2)), 'constant')
def segment(img):
# Search blank intervals.
img = _preprocess(img)
dots_per_col = np.apply_along_axis(lambda row: np.sum(row) // 255, 0, img)
blanks = []
was_blank = False
first_ch_x = None
prev_x = 0
x = 0
while x < CAPTCHA_WIDTH:
if dots_per_col[x] >= DOTS_THRESHOLD:
if first_ch_x is None:
first_ch_x = x
if was_blank:
# Skip first blank.
if prev_x:
blanks.append((prev_x, x))
# Don't allow too tight chars.
x += CH_MIN_WIDTH
was_blank = False
elif not was_blank:
was_blank = True
prev_x = x
x += 1
blanks = [b for b in blanks if b[1] - b[0] >= BLANK_THRESHHOLD]
# Add last (imaginary) blank to simplify following loop.
blanks.append((prev_x if was_blank else CAPTCHA_WIDTH, 0))
# Get chars.
chars = []
x1 = first_ch_x
widest = 0, 0
for i, (x2, next_x1) in enumerate(blanks):
width = x2 - x1
# Don't allow more than CH_WIDTH * 2.
extra_w = width - CH_WIDTH * 2
extra_w1 = extra_w // 2
extra_w2 = extra_w - extra_w1
x1 = max(x1, x1 + extra_w1)
x2 = min(x2, x2 - extra_w2)
ch = img[:CAPTCHA_HEIGHT, x1:x2]
y1 = find_filled_row(ch[::])
y2 = CAPTCHA_HEIGHT - find_filled_row(ch[::-1])
ch = ch[y1:y2]
chars.append(ch)
if width > widest[0]:
widest = x2 - x1, i
x1 = next_x1
# Fit chars into boxes.
chars2 = []
for i, ch in enumerate(chars):
widest_w, widest_i = widest
# Split glued chars.
if len(chars) < NUM_CHARS and i == widest_i:
ch1 = ch[:, 0:widest_w // 2]
ch2 = ch[:, widest_w // 2:widest_w]
chars2.append(pad_ch(ch1))
chars2.append(pad_ch(ch2))
else:
ch = ch[:, 0:CH_WIDTH]
chars2.append(pad_ch(ch))
assert len(chars2) == NUM_CHARS, 'bad number of chars'
return chars2
chars2 = segment(cv2.imdecode(img_array, cv2.IMREAD_GRAYSCALE))
fig = plt.figure()
for i, char in enumerate(chars2):
a = fig.add_subplot(1, 4, i+1)
plt.imshow(cv2.cvtColor(char, cv2.COLOR_GRAY2BGR))
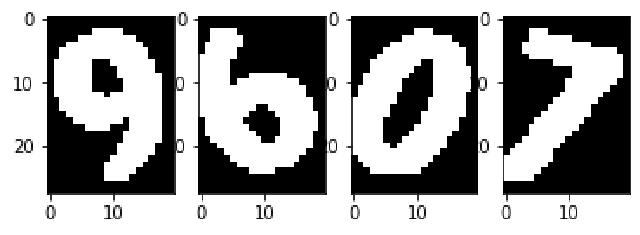
Other image-related processing functions
def check_image(img):
assert img is not None, 'cannot read image'
assert img.shape == (CAPTCHA_HEIGHT, CAPTCHA_WIDTH), 'bad image dimensions'
def read_image_file(fpath):
with open(fpath, 'rb') as f:
return decode_image(f.read())
def decode_image(data):
data = np.frombuffer(data, np.uint8)
img = cv2.imdecode(data, cv2.IMREAD_GRAYSCALE)
check_image(img)
return img
def get_ch_data(img):
data = img.flatten() & 1
assert len(data) == NUM_INPUT, 'bad data size'
return data
Neural Network and Training
# nn net define
NUM_INPUT = CH_WIDTH * CH_HEIGHT
NUM_NEURONS_HIDDEN = NUM_INPUT // 3
NUM_OUTPUT = 10
class Net(torch.nn.Module):
def __init__(self, n_feature, n_hidden, n_output):
super(Net, self).__init__()
self.hidden = torch.nn.Linear(n_feature, n_hidden) # hidden layer
self.out = torch.nn.Linear(n_hidden, n_output) # output layer
def forward(self, x):
x = F.relu(self.hidden(x)) # activation function for hidden layer
x = self.out(x)
return x
Start training based on the previously obtained character set
def train(captchas_dir):
net = Net(n_feature=NUM_INPUT, n_hidden=NUM_NEURONS_HIDDEN, n_output=NUM_OUTPUT)
optimizer = torch.optim.SGD(net.parameters(), lr=0.02, momentum=0.9)
loss_func = torch.nn.CrossEntropyLoss()
captchas_dir = os.path.abspath(captchas_dir)
captchas = glob.glob(captchas_dir + '/*.png')
x, y = [], []
for i, name in enumerate(captchas):
answer = re.match(r'.*(\d{4})\.png$', name)
if not answer:
continue
answer = answer.group(1)
fpath = os.path.join(captchas_dir, name)
try:
img = read_image_file(fpath)
ch_imgs = segment(img)
for ch_img, digit in zip(ch_imgs, answer):
x.append(get_ch_data(ch_img))
y.append(int(digit))
except Exception as e:
print('Error occured while processing {}: {}'.format(name, e))
else:
if (i + 1) % 25 == 0:
print('{}/{}'.format(i + 1, len(captchas)))
x, y = torch.from_numpy(np.array(x)).type(torch.FloatTensor), torch.from_numpy(np.array(y)).type(torch.LongTensor)
x, y = Variable(x), Variable(y)
for t in range(100):
out = net(x) # input x and predict based on x
loss = loss_func(out, y) # must be (1. nn output, 2. target), the target label is NOT one-hotted
optimizer.zero_grad() # clear gradients for next train
loss.backward() # backpropagation, compute gradients
optimizer.step() # apply gradients
return net
net = train(CAPTCHA_DIR)
25/400
50/400
75/400
100/400
125/400
150/400
175/400
200/400
225/400
250/400
275/400
300/400
325/400
350/400
375/400
400/400
print(net)
Net(
(hidden): Linear(in_features=560, out_features=186, bias=True)
(out): Linear(in_features=186, out_features=10, bias=True)
)
Predicting new figures
def predict(net, img_content):
def get_digit(ch_img):
x = torch.from_numpy(get_ch_data(ch_img)).type(torch.FloatTensor)
output = net(Variable(x))
_, predicted = torch.max(output.data, 0)
# return str(Variable(predicted).data[0])
return str(predicted.item())
img = decode_image(img_content)
ch_imgs = segment(img)
return ''.join(map(get_digit, ch_imgs))
img_content = get_captcha()
plt.imshow(plt.imread(BytesIO(img_content)))
result = predict(net, img_content)
plt.title(result)
Text(0.5, 1.0, '1707')
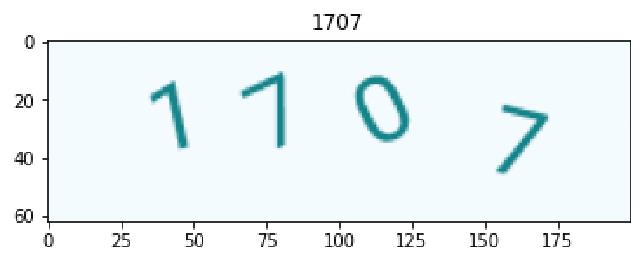
The complete program is included in captcha.py